
[image caption]
Research on predictive modeling informs mangrove conservation
Mangroves store high amounts of carbon in soil and vegetation. To better manage these carbon-rich ecosystems, scientists must accurately map where and how much carbon is stored within such coastal wetlands. However, it is unclear how accurate maps are relative to other forms of data, presenting a crucial information gap in the design of forest carbon programs.
Now, new research describes the importance of novel maps in guiding mangrove managers and policy-makers. The authors of a study, published in the journal Environmental Research Letters on July 23, 2020, compare estimates of mangrove carbon produced through predictive modeling, a technique using statistics and data science, against traditional approaches such as field-based measurements.
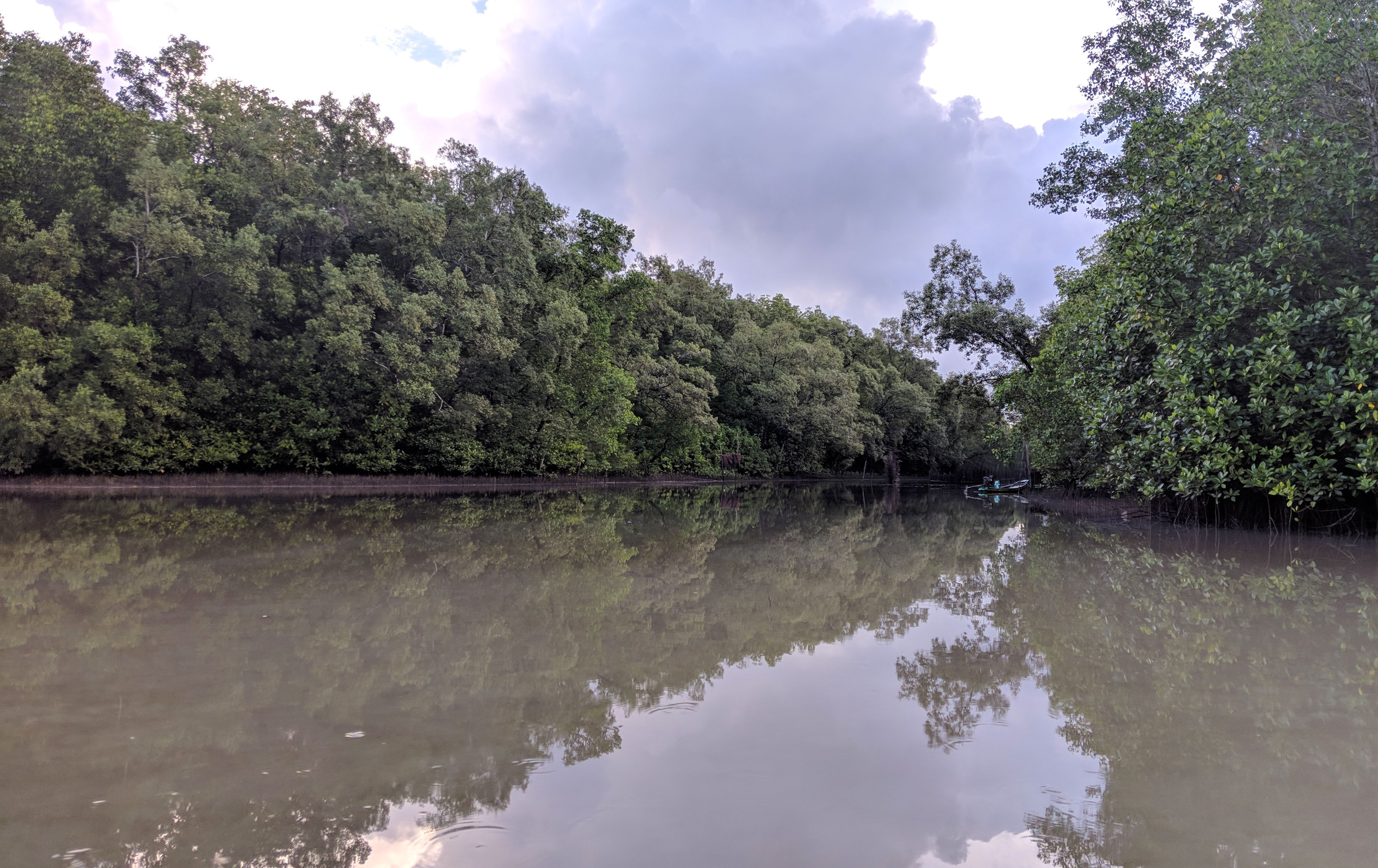
[image caption]
“We wanted to better understand the spatial scales at which global models of mangrove carbon align—or fail to align—with common methods for estimating carbon such as forest inventories,” explains study lead author Jacob Bukoski, a PhD candidate in the Department of Environmental Science, Policy and Management. “These modeled data are publicly available and are produced at relatively high spatial resolutions, which can be really valuable for people designing climate change mitigation programs in mangroves.”
Guidelines for the traditional measurement approaches are delineated by the Intergovernmental Panel on Climate Change (IPCC), the leading authority on carbon stock estimation. But because IPCC instructions on the use of modeled data are somewhat ambiguous, Bukoski and his team wanted to compare carbon stock estimates and assess the accuracy of the IPCC map data.
Using three different traditional methodological approaches from the IPCC, the authors estimated ecosystem level carbon stocks for mangrove sites in Brazil, the United Arab Emirates, and Thailand. The researchers then compared the values against model estimates for the same sites, and the modeled data out-performed the simplest IPCC methods. However, the predictive models were not definitively better than one IPCC method, which requires boots-on-the-ground measurements of trees and soil.
The findings have implications for carbon stock assessments. “Modeled data can improve your accuracy for very little cost. In fact, the data are free,” said Bukoski. “Whereas field inventories can be expensive to implement, using geographic information systems (GIS) software to analyze the model data can get you close to site-level estimates.”
Though both methods produce estimates rather than measurements, inherently subject to some uncertainty, the authors argue that the modeled data of mangrove carbon stocks are valuable. “Our hope is that the research will support NGOs and other programs which are working to conserve and restore mangroves,” according to Bukoski. “These programs often do not have the resources to run expensive field inventories, and our goal was to help provide guidance on how modeled datasets might help.”
The study, which can be found on the Environmental Research Letters website, was conducted with research from Angie Elwin, Rich A. Mackenzie, Sahadev Sharma, Joko Purbopuspito, Benjamin Kopania, Maybeleen Apwong, Roongreang Poolsiri, and Matthew D. Potts.